Clinical Data Management – Overview, Tools, and Best Practices
- October 18, 2024
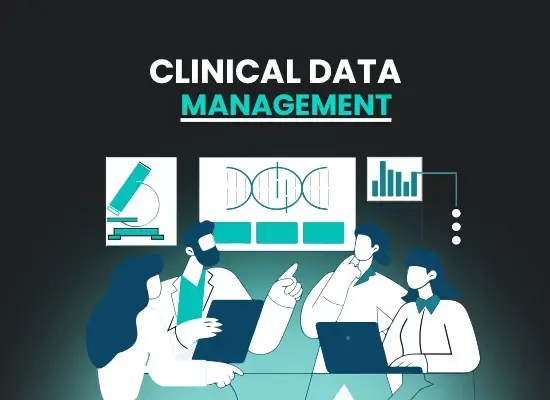
Table of Contents
Effective clinical data management enhances the quality of research and speeds up drug development. It helps researchers make informed decisions based on solid evidence. In this post, we will explore the key components of clinical data management, its importance in the healthcare industry, and best practices to implement for success. Dive into the world of clinical data management and discover how it can transform research outcomes.
Before we start, did you know that over 80% of clinical trials face delays due to poor data management? Clinical data management is crucial in ensuring accurate and reliable results in research. This process involves collecting, cleaning, and analyzing data to support clinical trials.
Key Takeaways:
- Understand the importance of clinical data management in ensuring accurate and reliable data for clinical trials, which is crucial for patient safety and effective treatment development.
- Recognize the key role of a clinical data manager, who oversees data collection, validation, and reporting, ensuring compliance with regulatory standards.
- Familiarize yourself with the various tools and software available for clinical data management to streamline processes and improve efficiency in data handling.
- Implement best practices in clinical research data management, such as maintaining clear documentation and regular audits to enhance data integrity.
- Stay informed about security and compliance measures necessary to protect sensitive patient information in clinical trials.
- Leverage real-world examples and case studies to learn from successful clinical data management strategies and avoid common pitfalls.
Getting to Know Clinical Data Management:
What is Clinical Data Management?
Clinical Data Management (CDM) is a vital part of clinical research. It ensures that the data collected during trials is accurate and reliable. CDM involves various processes like data collection, cleaning, and management. According to NCBI, these processes help researchers generate high-quality data.
Data collection starts with gathering information from clinical trial subjects. This includes their medical history, treatment details, and outcomes. Data can come from multiple sources such as questionnaires, lab tests, or electronic health records. The objective is to capture all relevant information that can impact the study results.

Once data is collected, it undergoes a cleaning process. This step identifies and corrects errors or inconsistencies in the data. For example, if a subject’s age is recorded incorrectly, this needs to be fixed before further analysis. Cleaning ensures the dataset is accurate and ready for statistical analysis.
Finally, data management involves organizing and storing the cleaned data securely. Researchers must comply with regulatory standards to protect participants’ privacy and ensure data integrity. Proper management allows for easy access and retrieval of data when needed.
Try it - Love it - Buy it
Avail Our Free Trial Now!
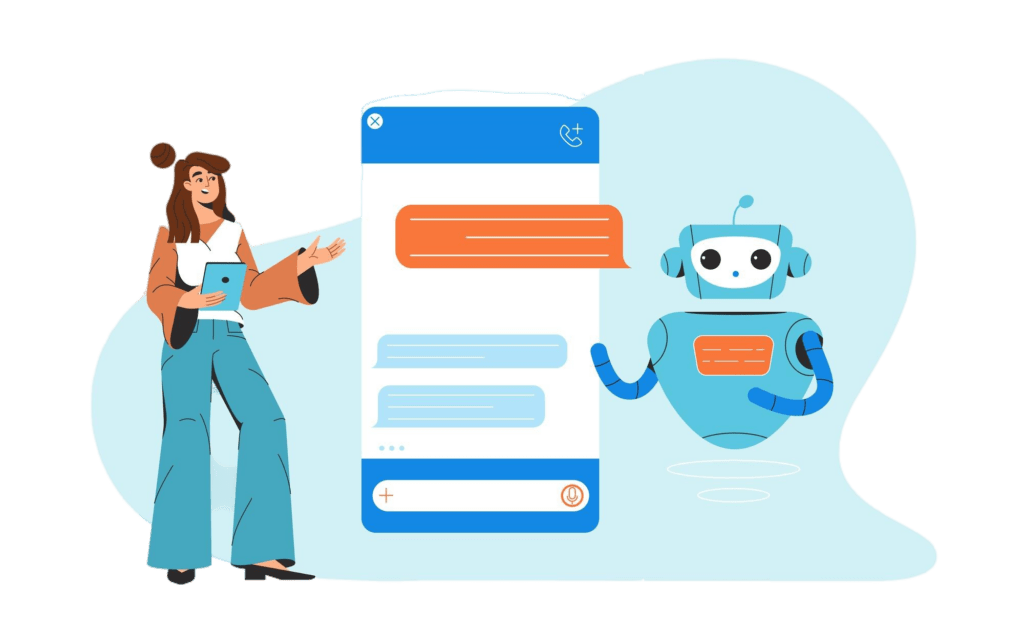
Why Data Management Matters in Clinical Research?
Data management plays a crucial role in the success of clinical trials. Team members involved in CDM are active throughout all stages of the trial. They start from the design phase and continue until completion. Their involvement ensures that data quality remains high from beginning to end.
The collection and management of subject data must comply with regulatory standards. These standards are set by organizations like the FDA or EMA. Compliance helps maintain the credibility of the study results. If data is not managed properly, it can lead to incorrect conclusions about a treatment’s effectiveness or safety.
High-quality data also builds trust with stakeholders. Sponsors, regulatory agencies, and medical professionals rely on accurate data to make informed decisions. Poorly managed data can result in delays or even halting of trials. This can have significant financial implications for companies involved in drug development.
Effective CDM leads to statistically sound results. Researchers use these results to support claims about new treatments or interventions. Without proper data management, studies may face challenges during peer review or approval processes.
The Essentials of Clinical Data:
Several essential elements contribute to effective clinical data management. First, having a clear plan for data collection is important. This plan outlines what information will be gathered and how it will be used. It sets expectations for team members involved in the trial.
Next, using technology can greatly enhance CDM processes. Electronic Data Capture (EDC) systems allow for real-time data entry and monitoring. These systems reduce human error and streamline the workflow for researchers. They also provide tools for tracking changes made to the dataset.
Training team members on best practices in CDM is crucial as well. Staff must understand how to collect, clean, and manage data effectively. Ongoing education can improve skills and keep everyone updated on regulatory changes.
Lastly, regular audits can help maintain high standards in CDM practices. Audits identify areas where improvements can be made and ensure compliance with regulations. They also reinforce a culture of accountability within research teams.
1- Data Quality:
Ensure robust validation processes to maintain high data integrity.
2- Regulatory Compliance:
Familiarize yourself with relevant regulations (e.g., FDA, EMA) to avoid legal issues.
3- Technology Infrastructure:
Assess the compatibility of existing systems with new data management tools.
4- Data Security:
Implement strong security measures to protect sensitive patient information.
5- Standardization:
Use standardized data formats and terminologies for consistency across studies.
6- Training and Expertise:
Ensure team members are well-trained in clinical data management practices and tools.
7- Data Accessibility:
Facilitate easy access to data for stakeholders while maintaining security protocols.
8- Timeliness:
Monitor timelines for data collection and analysis to meet project deadlines.
9- Collaboration Tools:
Utilize effective communication and collaboration platforms for seamless teamwork.
10- Cost Management:
Evaluate budget implications and seek cost-effective solutions without compromising quality.
The Role of a Clinical Data Manager:
What Is a Clinical Data Manager and What Does a Clinical Data Manager Do?
Clinical Data Managers (CDMs) play an important role in the healthcare industry. They gather and manage data from clinical trials. This data helps evaluate the safety and effectiveness of various treatments. Treatments can include drugs, medical devices, and digital health tools.
CDMs ensure that the data collected is accurate and reliable. They follow strict guidelines to maintain data quality. This process involves designing clinical trial database management and monitoring data entry. They also check for errors and inconsistencies. This attention to detail is crucial for making informed decisions in healthcare.
The work of a CDM directly impacts patient safety. For example, if a new drug is being tested, the CDM analyzes how well it works. They look at side effects and overall effectiveness. Their findings help determine if the drug is safe for public use.
Skills You Need to Be a Clinical Data Manager:
To succeed as a Clinical Data Manager, specific skills are essential. First, strong analytical skills are needed. CDMs must interpret complex data sets accurately. They often use statistical software to analyze trends and results.
Communication skills are also vital. CDMs work with different teams, including researchers and regulatory authorities. Clear communication ensures everyone understands the data findings and their implications.
Technical skills are important too. Proficiency in clinical trial database management is required. Familiarity with programming languages like SQL can be beneficial. These tools help CDMs manage large volumes of data effectively.
Problem-solving skills are necessary as well. Issues may arise during data collection or analysis. CDMs need to identify these problems quickly and find solutions.
How to Become a Clinical Data Manager?
Becoming a Clinical Data Manager typically requires formal education and experience in the field. Most CDMs hold a degree in life sciences or a related area. Courses in statistics or data analysis provide a solid foundation.
Gaining practical experience is crucial for aspiring CDMs. Internships or entry-level positions in clinical research can offer valuable insights into the role. Many professionals start as clinical trial assistants before advancing to CDM positions.
Certifications can enhance job prospects too. Organizations like the Society for Clinical Data Management offer certification programs. These certifications demonstrate expertise in clinical data management practices.
In the UK, entry-level Clinical Data Managers earn around £35,000 annually. With experience and added responsibilities, this figure can rise to about £42,500 per year. The average salary for a Clinical Data Manager is £35,177 per year.
Managing Data in Clinical Trials:
Management of Data in Clinical Trials:
Clinical trials generate a lot of data. This data needs proper management to ensure it is useful. Researchers collect information from various sources. This includes patient records, lab results, and survey responses. Each piece of data must be recorded accurately.
Data management begins with planning. A clear plan outlines how data will be collected and stored. It defines roles for the clinical data manager and the research team. They work together to make sure everyone knows their responsibilities. This teamwork helps avoid errors.
During the trial, data collection happens in real-time. Data managers monitor this process closely. They ensure that all information is entered correctly into the system. Any discrepancies should be addressed immediately. Missing or incorrect data can lead to issues later on.
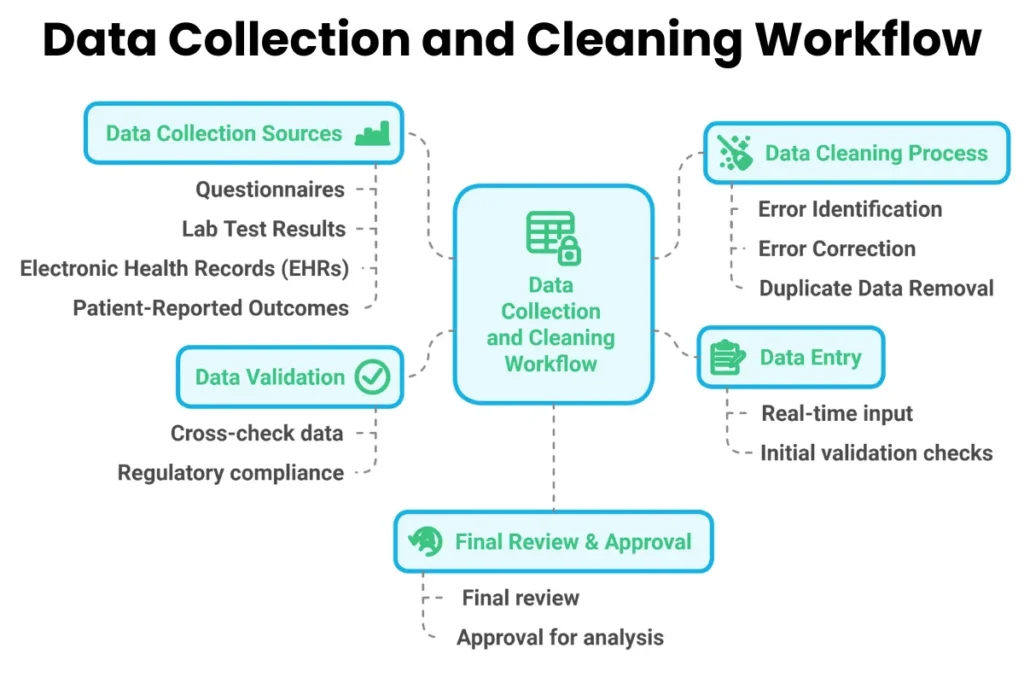
How to Handle Clinical Trial Database Management?
Handling a clinical trial database management requires specific steps. First, choose the right clinical trial database management system. The system should fit the trial’s needs. Some systems are designed for small studies, while others handle large-scale trials.
Next, establish a clear structure for the database. This includes defining fields for different types of data. For example, demographic information may include age, gender, and medical history. Defining these fields early makes data entry easier.
Training staff on how to use the clinical trial database management is crucial. Everyone involved must know how to enter and retrieve data properly. Regular training sessions help keep everyone updated on best practices.
Data security is another important aspect of clinical trial database management. Protecting sensitive patient information is essential. Organizations must comply with regulations like HIPAA in the U.S. These rules safeguard personal health information.
Lastly, regular backups are necessary. Backing up data prevents loss due to technical issues or human error. Establish a schedule for backups to ensure no data is left unprotected.
Ensuring Data Quality and Validation:
Ensuring high-quality data is vital in clinical trials. Quality data leads to reliable results and conclusions. One way to ensure quality is through validation checks during data entry.
Validation checks can identify errors before they become problems. For example, if someone enters an age that is not realistic, the system can flag it for review. These checks save time by catching mistakes early.
Another method to maintain quality is conducting audits regularly. Audits involve reviewing the collected data against original sources like patient records. This process helps verify that everything matches up correctly.
Training staff on best practices also contributes to quality assurance. Team members should understand the importance of accurate data entry and reporting. Encouraging open communication among team members helps address concerns quickly.
Tools and Software for Clinical Trial Data Management:
An Overview of Clinical Trial Data Management Software:
Clinical trial data management or clinical data management (CDM) software plays a vital role in clinical trials. It helps researchers collect, manage, and analyze data efficiently. These AI medical products and tools ensure that the data gathered is accurate and reliable.
Various software solutions exist to meet the needs of different studies. They help streamline processes and maintain compliance with industry standards. Following guidelines is critical in CDM. These guidelines ensure the integrity of trial data and protect patient safety.
The software typically includes features like data entry, validation, and reporting. Users can track patient information and monitor study progress easily. This enhances communication between team members and improves overall efficiency.
What to Look for in a Clinical Data Management System?
Choosing the right clinical data management system requires careful consideration. Several key factors should guide your decision-making process.
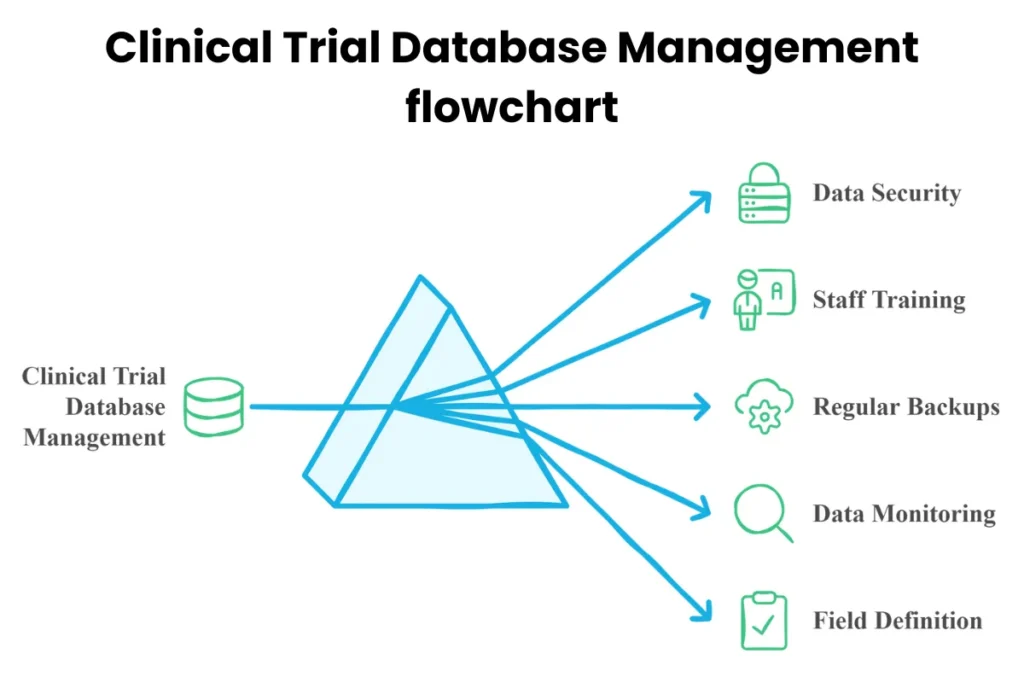
1- User-Friendliness:
The software must be easy to navigate. Complex systems can lead to errors during data entry.
2- Data Security:
Patient privacy is paramount. Look for systems that offer strong security measures to protect sensitive information.
3- Integration Capabilities:
The software should integrate well with other systems used in the trial. This includes electronic health records (EHR) and laboratory information systems (LIS).
4- Regulatory Compliance:
Ensure that the software complies with regulations set by authorities like the FDA and EMA. This helps avoid legal issues down the line.
5- Support and Training:
Good customer support is essential. Training resources can help staff use the system effectively.
6- Cost-Effectiveness:
Budget constraints are common in clinical trials. Compare costs of different systems while considering their features.
By evaluating these factors, teams can select a system that meets their specific needs.
Top Clinical Data Management Tools You Should Know:
Several prominent tools dominate the clinical data management landscape. Each tool offers unique features tailored to various research requirements.
1- ORACLE CLINICAL:
This tool provides comprehensive solutions for managing clinical trials. It supports complex studies and ensures regulatory compliance.
2- CLINTRIAL:
Known for its flexibility, CLINTRIAL allows customization based on specific project needs. It simplifies data collection and monitoring.
3- MACRO:
MACRO focuses on usability and efficiency. Its intuitive interface makes it easier for users to enter and manage data accurately.
4- RAVE:
RAVE is widely recognized for its robust capabilities in real-time data access and analytics. It helps researchers make informed decisions quickly.
5- eClinical Suite:
This all-in-one platform integrates various functions such as data capture, management, and reporting. It streamlines workflows throughout the trial process.
6- Smart Medical History:
Smart Medical History utilizes advanced artificial intelligence to efficiently collect and analyze patient medical histories, ensuring high data accuracy and streamlining the clinical trial management process.
These tools are essential in maintaining high-quality data throughout clinical trials. They help researchers adhere to guidelines while ensuring efficient study management.
Tool/Software | Description | Key Features | Ideal For |
---|---|---|---|
REDCap | A secure web application for building and managing online surveys and databases. | User-friendly interface, project management, audit trails | Academic research, clinical trials |
Oracle Siebel CTMS | Comprehensive clinical trial management system. | Workflow automation, data integration, reporting tools | Large pharmaceutical companies |
Medidata Solutions | Cloud-based platform for clinical trials management. | Real-time data access, analytics, patient engagement tools | Biotech firms, CROs |
OpenClinica | Open-source software for managing clinical trials. | Customizable forms, data validation, compliance tracking | Small to mid-sized organizations |
Veeva Vault | Cloud-based content management system for life sciences. | Document management, collaboration tools, regulatory compliance | Life science organizations |
Castor EDC | Electronic data capture solution for clinical research. | User-friendly interface, integration capabilities, multi-language support | Researchers and clinicians |
ClinCapture | Flexible electronic data capture software. | Customizable forms, real-time data access, compliance features | Clinical researchers |
TrialMaster | Clinical trial management software with data capture capabilities. | Real-time monitoring, reporting tools, user-friendly design | Research institutions |
IBM Watson Health | AI-driven analytics and clinical data management platform. | Predictive analytics, patient insights, integration with EHRs | Healthcare providers |
Statistix | Statistical analysis software for clinical research. | Comprehensive statistical tests, graphical output, user-friendly interface | Statisticians and researchers |
Clinical Data Management Services:
Outsourcing Clinical Data Management to a CRO:
Many companies choose to outsource clinical data management to a Contract Research Organization (CRO). This decision often comes from the need for specialized expertise. CROs have trained professionals who focus on collecting, analyzing, and managing clinical trial data. They understand the regulations and standards required in this field.
Outsourcing can save time and money. For example, a pharmaceutical company may lack the resources to handle large datasets. By partnering with a CRO, they can access advanced tools and skilled staff. This allows them to concentrate on their core business activities while ensuring quality data management.
In 2020, reports showed that over 60% of clinical trials were outsourced to CROs. This trend continues as more organizations recognize the advantages. By outsourcing, companies can also scale operations easily. They can adjust the level of service based on project needs without hiring full-time employees.
The Benefits of Clinical Data Management Services:
Clinical data management services offer several key benefits. First, they ensure high-quality data collection. Good data is crucial for making informed decisions in clinical trials. Accurate data helps researchers draw valid conclusions about new treatments.
Second, these services improve efficiency. Well-organized data management processes speed up the trial timeline. For instance, automated systems reduce manual errors and streamline workflows. As a result, studies can move forward faster.
Third, compliance with regulatory requirements is essential for data management in clinical research and for clinical research. Data management services help maintain adherence to guidelines set by organizations like the FDA or EMA. These regulations protect patient safety and ensure reliable results.
Other benefits include:
- Access to advanced technology
- Expertise in specific therapeutic areas
- Cost-effectiveness compared to in-house management
Choosing the Right Clinical Data Management CRO:
Selecting the right CRO for clinical data management is critical. Companies should consider several factors during this process. First, evaluate the CRO’s experience in similar projects. A proven track record boosts confidence in their capabilities.
Next, assess their technology and tools. The right software can enhance data integrity and security. It’s important that the CRO uses modern systems compatible with your needs.
Communication is another vital aspect. Clear communication leads to better collaboration between teams. Ensure that the CRO has a responsive support system for addressing questions or concerns.
Finally, consider costs involved with their services. While it’s tempting to choose the cheapest option, quality should not be compromised. Balancing cost and value is essential for successful partnerships.
Best Practices in Clinical Research Data Management:
Data Management in Clinical Research:
Data management is crucial in clinical research. It ensures that data is accurate and reliable. Researchers collect vast amounts of information during trials. This can include patient details, treatment responses, and side effects. Organizing this data effectively helps in drawing meaningful conclusions.
First, it’s essential to establish a data collection plan. This plan outlines what data to collect and how to do it. It should detail the methods for gathering information from participants. For example, using electronic data capture (EDC) systems can streamline this process. These systems allow researchers to input data directly into a database. This reduces errors that may occur with paper forms.
Next, regular audits are necessary. Audits help identify any discrepancies or issues early on. They also ensure that the data remains consistent throughout the study. A well-structured protocol can guide these audits, making sure all team members understand their roles.
Finally, training staff is vital. All personnel involved in data collection must know best practices. Training should cover topics such as data entry, handling sensitive information, and understanding protocols. Well-trained staff contributes to high-quality data management.
Keeping Your Data Safe and Secure:
Security is a top priority for data management in clinical research and clinical research data management itself. Protecting patient information is not just ethical; it’s also a legal requirement. Organizations must comply with regulations like HIPAA in the United States. These laws govern how personal health information should be handled.
Implementing strong security measures is essential. This includes using encryption for stored data and during transmission. Encryption makes information unreadable to unauthorized users. Access controls should limit who can view or modify the data. Only authorized personnel should have access to sensitive information.
Regularly updating software and systems also plays a role in security. Cyber threats evolve constantly, so keeping systems current is crucial. Software updates often include security patches that protect against new vulnerabilities.
Conducting risk assessments helps identify potential weaknesses in your system. By evaluating risks, organizations can take proactive steps to mitigate them. This may involve enhancing physical security at data storage locations or increasing cybersecurity measures.
Meeting Regulatory Standards in Data Management:
Compliance with regulatory standards is non-negotiable in clinical research data management. Regulatory bodies set strict guidelines for how data should be collected, stored, and reported. Meeting these standards ensures the integrity of the research process.
Understanding regulations such as Good Clinical Practice (GCP) is fundamental. GCP provides a framework for conducting clinical trials ethically and scientifically soundly. Adhering to these guidelines helps protect participant safety and ensures valid results.
Documentation is another critical aspect of compliance. All processes related to data management must be thoroughly documented. This includes protocols for data collection, storage procedures, and audit trails. Proper documentation supports transparency and accountability.
Regular training sessions on regulatory requirements keep staff informed about changes in laws or guidelines. Staying updated helps avoid non-compliance issues that could jeopardize a study’s validity.
Advanced Topics in Clinical Trial Data Management:
New Innovations in Clinical Trial Data Management:
Recent years have seen significant advancements in clinical trial data management. These innovations improve how researchers collect, store, and analyze data. Electronic data capture (EDC) systems are now common. They allow for real-time data entry during clinical trials. This reduces errors and speeds up the process.
Cloud technology has also transformed data management. It provides secure storage solutions. Researchers can access data from anywhere at any time. This flexibility enhances collaboration among teams spread across different locations.
Mobile applications play a crucial role too. They enable patients to input their health information directly. This leads to more accurate and timely data collection. Wearable devices track patient metrics continuously. This offers researchers valuable insights into patient health during trials.
What’s Next for Clinical Data Management Systems?
The future of clinical data management systems looks promising. Integration of advanced technologies will be key. Systems will likely incorporate blockchain technology for enhanced security. Blockchain can ensure that data is tamper-proof and transparent.
Another trend is the shift towards patient-centric approaches. Systems will focus more on user experience. Intuitive interfaces will make it easier for researchers and participants to use these systems effectively.
Regulatory compliance will remain a priority. As regulations evolve, systems must adapt quickly. Future systems need to streamline compliance processes without sacrificing quality or accuracy.
Using Artificial Intelligence in Data Management:
Artificial intelligence (AI) is making waves in clinical data management. AI algorithms can analyze large datasets quickly. They can identify patterns that humans might miss. This capability accelerates the research process significantly.
Predictive analytics is one area where AI shines. It helps researchers forecast outcomes based on historical data. This allows for better planning and resource allocation during trials.
AI also assists with data cleaning and validation. It automatically flags inconsistencies in the data collected. This reduces the burden on human staff and speeds up the overall workflow.
Using Artificial Intelligence in Data Management:
Case Study | Organization | Focus Area | Key Findings | Impact on Clinical Data Management |
---|---|---|---|---|
Clinical Trial for Diabetes | XYZ Pharmaceuticals | Diabetes Drug Development | Improved data accuracy through real-time monitoring. | Enhanced patient safety and regulatory compliance. |
Oncology Research Project | ABC Cancer Institute | Cancer Treatment Efficacy | Identified biomarkers for treatment response. | Streamlined data collection and analysis processes. |
Vaccine Development Initiative | Global Health Organization | COVID-19 Vaccine Trials | Rapid data sharing led to accelerated approval. | Established new standards for data transparency and collaboration. |
Heart Disease Study | DEF Medical Center | Cardiovascular Risk Factors | Correlation between lifestyle changes and outcomes. | Integrated patient-reported outcomes into data management systems. |
Mental Health Intervention | GHI Behavioral Health | Depression Treatment | Effective use of mobile apps for data collection. | Increased engagement and retention in clinical trials. |
Rare Disease Registry | JKL Rare Diseases Network | Genetic Disorders | Comprehensive data repository improved diagnosis rates. | Facilitated collaboration among researchers and clinicians. |
Longitudinal Aging Study | MNO Aging Institute | Elderly Population Health | Identified trends in health decline over time. | Informed public health strategies and resource allocation. |
Drug Repurposing Research | PQR Pharma Solutions | Existing Drug Applications | Successful identification of new uses for old drugs. | Optimized resource utilization in clinical trials. |
Digital Health Intervention | STU Tech Innovations | Remote Patient Monitoring | Improved adherence through digital tools. | Revolutionized patient data collection methods. |
Pediatric Clinical Trials | VWX Children’s Hospital | Pediatric Medicine | Unique challenges in data management for minors. | Developed specialized protocols for ethical data handling. |
Successful Clinical Study Data Management Stories:
A successful clinical study in 2019 involved a new diabetes medication. Researchers collected data from over 1,000 participants across multiple sites. They used electronic data capture (EDC) systems to streamline the process. This approach reduced errors and improved data quality. The study finished on time, leading to quicker results.
Another example comes from a cancer treatment trial in 2020. This study focused on a targeted therapy for breast cancer. The team implemented robust data management practices. They ensured real-time access to data for all stakeholders. As a result, they identified trends faster and made timely adjustments. This led to more effective patient monitoring and improved outcomes.
In 2021, a vaccine trial showcased the importance of data management. Researchers faced an urgent timeline due to the pandemic. They utilized cloud-based systems for data storage and sharing. This allowed teams to collaborate seamlessly across different locations. The quick access to accurate data sped up decision-making processes significantly.
Overcoming Challenges in Clinical Data Management:
Clinical data management often presents challenges. One significant issue is dealing with large volumes of data. In many studies, thousands of data points are generated daily. Managing this information can overwhelm traditional systems. To tackle this, many organizations turned to automation tools. These tools help in organizing and analyzing large datasets efficiently.
Another challenge in clinical study data management, is ensuring compliance with regulations. Regulatory bodies like the FDA set strict guidelines for data handling. Meeting these requirements can be complex and time-consuming. Organizations must train staff thoroughly on these regulations. Regular audits also help maintain compliance and improve processes.
Data security for clinical study data management remains a critical concern as well. With increasing cyber threats, protecting sensitive information is vital. Many organizations adopt advanced encryption methods to safeguard data. They also conduct regular security assessments to identify vulnerabilities. These steps ensure that patient information remains confidential and secure.
What We’ve Learned from Clinical Data Management Projects?
Numerous lessons emerge from clinical data management projects. First, early planning is essential for success. Teams must define their goals and select appropriate tools upfront. This clarity helps avoid delays later in the project.
Collaboration plays a crucial role as well. Engaging all stakeholders early fosters better communication. It ensures everyone understands their responsibilities and timelines. This teamwork leads to smoother operations throughout the study.
Lastly, adaptability is key in clinical data management. Smart Medical History is an AI that provides the most secure data management. Projects including clinical study data management often face unexpected changes or challenges. Teams that embrace flexibility can pivot quickly when needed. For instance, during the COVID-19 pandemic, many studies shifted to remote monitoring solutions.
Security and Compliance for Clinical Data Management:
Policy Name | Description | Compliance Standards | Responsible Parties |
---|---|---|---|
Data Privacy Policy | Guidelines on handling personal health information. | HIPAA, GDPR | Data Managers, IT Security |
Data Integrity Policy | Ensures accuracy and consistency of clinical data. | FDA 21 CFR Part 11 | Clinical Data Managers |
Access Control Policy | Defines user access levels to clinical data systems. | ISO/IEC 27001 | IT Security Admin |
Data Retention Policy | Outlines retention periods for clinical data records. | FDA GCP | Compliance Officers |
Incident Response Policy | Procedures for responding to data breaches or incidents. | NIST SP 800-61 | IT Security, Compliance Team |
Audit Trail Policy | Requirements for maintaining records of data changes. | FDA 21 CFR Part 11 | Clinical Data Managers |
Training and Awareness Policy | Mandates training on compliance and data security. | HIPAA, GCP | HR, Compliance Officers |
Vendor Management Policy | Guidelines for assessing third-party vendors’ compliance. | ISO 27001 | Procurement, Compliance Team |
Data Sharing Policy | Rules for sharing clinical data with external parties. | HIPAA, GDPR | Clinical Operations |
Risk Management Policy | Framework for identifying and mitigating data risks. | ISO 31000 | Risk Management Team |
Importance of Security:
Security plays a crucial role in clinical data management. Organizations must protect sensitive patient information. Breaches can lead to serious consequences, including loss of trust and legal penalties.
In 1996, the Health Insurance Portability and Accountability Act (HIPAA) established standards for protecting health information. HIPAA requires organizations to implement safeguards for electronic health records. These measures include access controls, encryption, and audit trails.
Effective security measures also help maintain data integrity. Unprotected data can be altered or deleted. This can jeopardize research outcomes and patient safety. Therefore, organizations must prioritize security protocols when managing clinical data.
Compliance Requirements:
Compliance with regulations is essential in clinical data management. Organizations must follow federal and state laws. These laws ensure that patient information remains confidential and secure.
HIPAA permits different levels of anonymization based on the sensitivity of the clinical dataset. For example, fully anonymized data does not identify individuals. This allows researchers to use the information without violating privacy rights. However, partially anonymized data may still contain identifiable elements. Organizations must handle such data with extra care.
Moreover, compliance involves regular audits. Audits assess whether an organization meets regulatory requirements. They help identify potential vulnerabilities in data management practices. Regular training for staff members on compliance is also vital. Employees should understand the importance of following protocols.
Best Practices for Security and Compliance:
Organizations should adopt best practices to enhance security and compliance in clinical data management. Implementing strong passwords is a fundamental step. Passwords should be complex and changed regularly.
Access controls are another critical measure. Only authorized personnel should have access to sensitive data. Role-based access limits exposure to necessary information only.
Data encryption is essential for protecting sensitive information during transmission and storage. Encrypted data is unreadable to unauthorized users. This significantly lowers the risk of breaches.
Regular software updates also play a key role in maintaining security. Outdated software can have vulnerabilities that hackers exploit. Keeping systems updated ensures they are protected against new threats.
Consequences of Non-Compliance:
Non-compliance with security regulations can result in severe penalties. Organizations may face hefty fines from regulatory bodies like the Office for Civil Rights (OCR). In some cases, non-compliance can lead to criminal charges against responsible individuals.
Beyond financial penalties, non-compliance can damage an organization’s reputation. Patients may lose trust in healthcare providers that fail to protect their information. This loss of trust can affect patient retention and overall business success.
Real-world examples illustrate these consequences well. In 2015, a major health insurer suffered a significant breach due to poor security measures. Millions of patient records were compromised, leading to a $16 million settlement with OCR.
Future Trends in Security:
The future of clinical data management will see advancements in security technology. Artificial intelligence (AI) will play a significant role in identifying threats faster than human analysts can detect them. AI tools can analyze patterns and flag unusual activities within datasets.
Blockchain technology is another promising development for securing clinical data management. Blockchain provides a decentralized way to store information securely. It ensures that any changes made to the data are recorded transparently.
As regulations evolve, organizations must stay informed about changes in compliance requirements. Continuous education on security practices will be essential for all staff members involved in clinical data management.
Resources and Tools for Clinical Data Managers:
Training and Certifications for Clinical Data Managers:
Clinical data management requires specialized knowledge. Many professionals pursue certifications to enhance their skills. The Society for Clinical Data Management (SCDM) offers the Certified Clinical Data Manager (CCDM) credential. This certification validates expertise in data management principles.
Training programs are also available. These programs cover essential topics like data collection, database design, and regulatory compliance. Some universities offer degrees specifically in clinical research or data management. Online courses provide flexibility for busy professionals.
Staying updated is crucial. Regulations change frequently in clinical trials. Continuous education helps data managers remain compliant with industry standards. Workshops and webinars are great resources for learning about new tools and techniques.
Must-Have Tools for Effective Data Management:
Data managers rely on various tools to streamline their work. Electronic Data Capture (EDC) systems are essential. They allow real-time data entry during clinical trials. Popular EDC systems include Medidata Rave and Oracle’s Siebel CTMS.
Data analysis software is another important tool. Programs like SAS and R help analyze large datasets efficiently. These tools can identify trends and ensure data quality.
Project management software aids in organization. Tools like Microsoft Project or Trello help track progress and deadlines. They keep teams aligned and informed throughout the study.
Data visualization tools also play a key role. Software like Tableau can turn complex data into easy-to-understand visuals. This makes it easier to present findings to stakeholders.
Finding Support and Community as a Clinical Data Professional:
Networking is vital for clinical data managers. Joining professional organizations provides access to valuable resources. Organizations like SCDM offer forums, conferences, and workshops for networking opportunities.
Online communities can also be helpful. Social media platforms like LinkedIn host groups focused on clinical data management. Members share experiences, tips, and job leads.
Mentorship programs connect less experienced professionals with seasoned experts. These relationships foster growth and development in the field. Mentors can provide guidance on navigating challenges within the industry.
Subscribe to our Newsletter!
Subscribe our Newsletter to stay updated
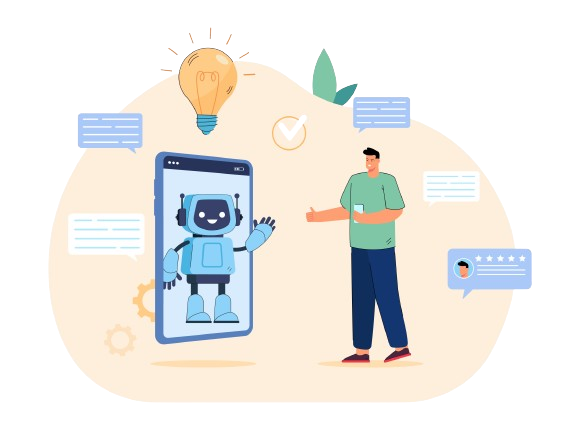
Closing Thoughts:
Clinical data management is not only crucial, but also necessary for successful clinical trials. It ensures data integrity, security, and compliance while streamlining processes. By understanding the roles, tools, and best practices, you can enhance your research outcomes.
Embrace the insights shared here to elevate your clinical management strategies. Stay informed about the latest tools and trends to boost your efficiency. Remember, effective data management not only protects your research but also builds trust with stakeholders. Dive deeper into resources available to you and take action today. Your next breakthrough could be just a click away!
Frequently Asked Questions (FAQs)
What is clinical data management?
Clinical data management (CDM) is the process of collecting, cleaning, and managing data from clinical trials to ensure accuracy and integrity. It supports regulatory compliance and enhances the reliability of study results.
What is a Clinical Data Manager?
A clinical data manager is a professional who oversees the collection, validation, and analysis of data in clinical trials to ensure its accuracy, integrity, and compliance with regulatory standards.
Why is a Clinical Data Manager important?
A Clinical Data Manager oversees data collection and ensures that it meets regulatory standards. Their expertise helps maintain data quality, which is crucial for successful clinical trials and accurate outcomes.
What tools are commonly used in clinical data management?
Popular tools include electronic data capture (EDC) systems, clinical trial management systems (CTMS), and statistical analysis software. These tools streamline data collection, storage, and analysis processes.
What are best practices in clinical research data management?
Best practices include maintaining data integrity, ensuring clear documentation, performing regular audits, and training staff on protocols. These practices enhance reliability and facilitate compliance with regulations.
How does security play a role in clinical data management?
Security is vital in CDM to protect sensitive patient information. Implementing robust security measures safeguards data against breaches and ensures compliance with regulations like HIPAA.
What services do Clinical Data Management companies offer?
CDM companies provide services such as data collection, validation, database design, statistical analysis, and reporting. These services help streamline clinical trials and improve efficiency.
Can you give an example of a real-world application of CDM?
In a recent diabetes drug trial, effective CDM helped identify data discrepancies early. This proactive approach ensured timely corrections, leading to reliable results that supported FDA approval.
Read More Blogs
See what’s trending in the medical world with our blogs.