Predictive Analytics in Healthcare: How it Improves Care and Reduce Risks
- December 12, 2024
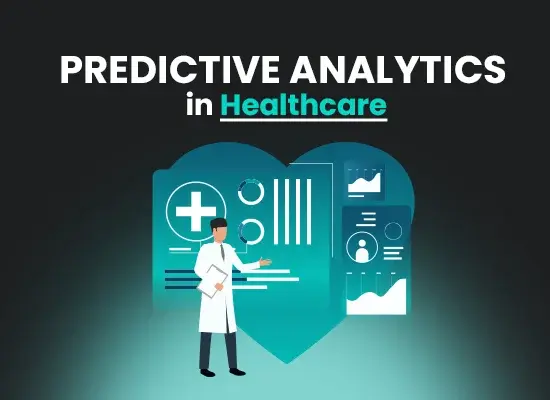
Table of Contents
In the past, healthcare decisions were largely based on experience and intuition. With predictive analytics, medical professionals can rely on data-driven insights to predict disease outbreaks, identify patients at risk, and improve treatment strategies. The key goal is to improve patient outcomes by anticipating health issues before they become critical.
Introduction to Predictive Analytics in Healthcare:
Predictive analytics in healthcare involves using data to make predictions about future health outcomes. It uses historical data, statistical algorithms, and machine learning techniques to identify trends, patterns, and potential risks.
What is Predictive Analytics in Healthcare?
Predictive analytics in healthcare is the practice of using data to forecast future health conditions and trends. It involves collecting data from various sources, such as patient medical records, lab results, and demographic information. This helps doctors, nurses, and other healthcare workers to anticipate and plan for future health events.
- Predicting which patients are at higher risk for certain diseases
- Identifying possible complications before they arise
For example, predictive analytics can help identify patients at high risk of heart disease based on their medical history, lifestyle factors, and genetic predispositions.
Why Predictive Analytics Matters in Healthcare?
Predictive analytics helps healthcare professionals make better decisions by providing data-based insights. It can improve both individual patient care and the overall healthcare system.
- Early identification of at-risk patients
- Reducing emergency room visits and hospital readmissions
For instance, hospitals use predictive models to anticipate patient needs during flu season. They can predict the number of patients likely to be admitted for flu-related issues, helping the hospital prepare in advance.
Try it - Love it - Buy it
Avail Our Free Trial Now!
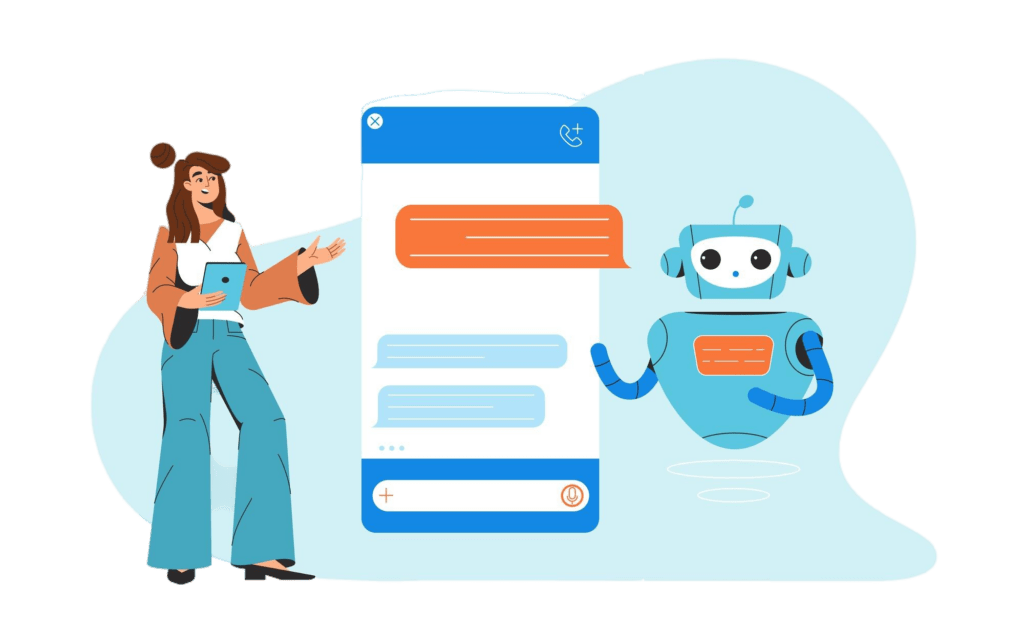
Predictive Analytics in Healthcare Using Big Data:
Big data refers to the vast amount of health-related information collected from various sources like medical records, patient surveys, wearable devices, and social media. Predictive analytics uses big data to uncover hidden patterns, predict health risks, and improve care delivery.
- Analyzing large datasets to detect trends in patient health
- Using data from wearable devices to monitor chronic conditions
For example, data from smartwatches and fitness trackers can be used to monitor a patient’s heart rate, sleep patterns, and physical activity. This information can help doctors predict potential health risks long before symptoms appear.
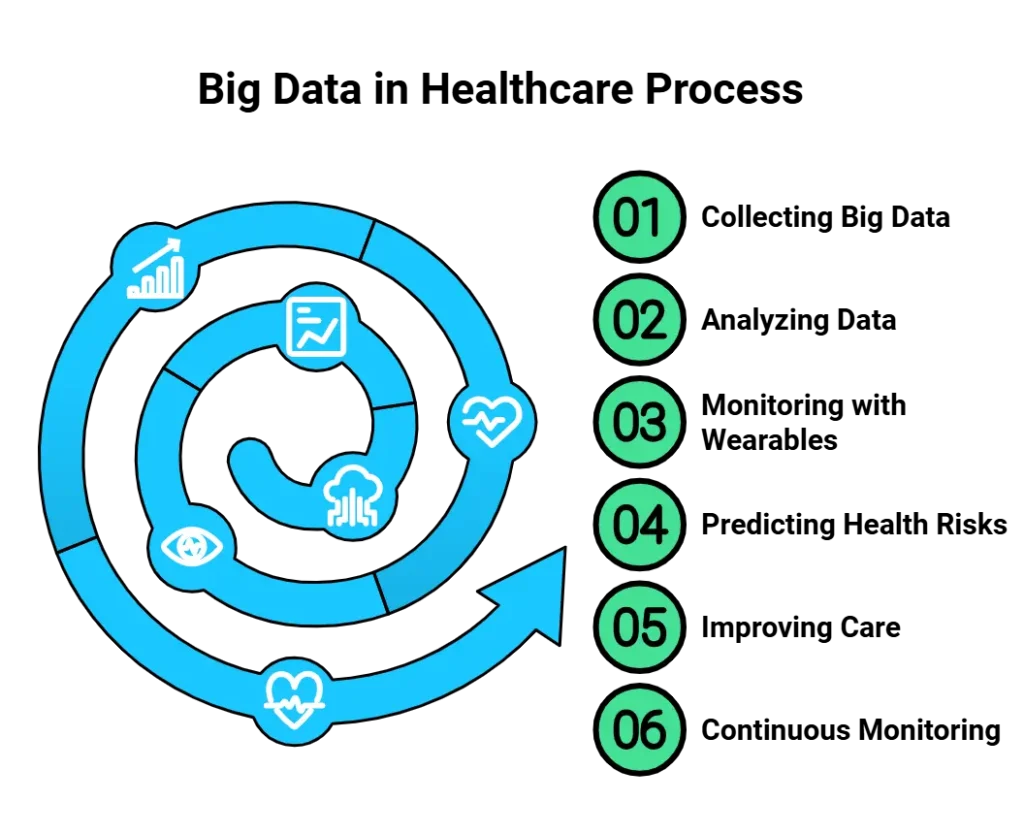
How Predictive Analytics is Changing Healthcare:
Predictive analytics is making a big difference in healthcare by using data to help predict patient outcomes and improve care. This approach looks at past health data to find patterns that can help doctors and healthcare workers make better decisions.
By predicting things like who might get sick or who needs extra care, predictive analytics can help healthcare systems get ahead of potential problems, leading to better patient care and fewer emergencies. The healthcare predictive analytics market is projected to expand at a compound annual growth rate (CAGR) of 27.67% through 2032.
Benefits of Predictive Analytics in Healthcare:
- Early detection of diseases: Predictive models can help spot signs of diseases before they become serious, allowing for earlier treatment.
- Improved patient care: Healthcare providers can use predictions to offer more timely and accurate care plans.
- Better resource management: Predictive analytics can help hospitals better plan for patient volume and staffing needs.
Cost savings: By preventing hospital readmissions and unnecessary tests, hospitals can save money while providing better care.
Importance of Predictive Analytics in Healthcare:
Predictive analytics is crucial in healthcare as it helps healthcare providers forecast potential health problems before they become serious. 66 percent of US healthcare organizations currently use predictive analytics.
Predictive models can identify chronic conditions early by analyzing patterns in patient data, such as medical history, lifestyle choices, and test results.
1- Early detection of health risks:
Predictive analytics helps identify potential health problems, such as heart disease or diabetes, before they become severe, allowing for timely medical intervention.
2- Better treatment planning:
By analyzing patient data, healthcare providers can anticipate complications or relapses, leading to more accurate and effective treatment plans.
3- Patient outcomes:
Early intervention based on predictive models often results in better health outcomes, as conditions can be managed or treated before they worsen, improving survival rates.
4- Enhanced resource management:
Predictive analytics helps hospitals forecast patient demand, allowing them to allocate staff, equipment, and beds more effectively, ensuring resources are available.
5- Reduced hospital readmissions:
By identifying patients at high risk of readmission, predictive models enable healthcare providers to offer preventive care, reducing the likelihood of patients.
6- Lower healthcare costs:
By predicting and preventing complications or hospital readmissions, predictive analytics can reduce the need for expensive treatments and hospital stays.
Examples of Predictive Analytics in Healthcare:
Predictive analytics is already being used in many healthcare settings with great success.
Predictive analytics in healthcare supports drug discovery, clinical trials, and personalized medicine, with related expenditures expected to reach USD 2 billion annually by 2025.
1- Emergency Room:
Predictive models are used to forecast ER patient volume, helping hospitals manage resources and reduce wait times efficiently.
2- Predicting Hospital Readmissions:
Hospitals use predictive models to identify patients at high risk of being readmitted, allowing for targeted interventions to prevent unnecessary returns.
3- Medication Dosage:
Predictive analytics helps determine the optimal dosage of medications based on patient characteristics, improving treatment outcomes and reducing side effects.
4- Predicting Mental Health Crises:
Predictive models analyze patient data to identify those at risk of mental health crises, allowing healthcare providers to offer timely support and prevent severe episodes.
5- Early Detection of Cancer
Predictive analytics is used to analyze medical imaging and genetic data to identify potential signs of cancer in its early stages, improving outcomes through early intervention.
6- Surgery Scheduling:
Predictive analytics helps hospitals forecast surgery demand, optimizing operating room schedules and reducing wait times for patients in need of surgical procedures
7- Patient Deterioration:
Predictive models analyze vital signs and health data to identify patients at risk of deterioration, enabling healthcare providers to intervene early and prevent adverse outcomes.
How to Use Predictive Analytics in Healthcare:
Healthcare organizations can use this tool to improve patient care, optimize operations, and predict medical trends. By analyzing patient records, treatments, and outcomes, predictive analytics provides valuable insights. It also helps boost patient retention by identifying factors affecting patient satisfaction and allowing proactive improvements to care.
Key Techniques in Healthcare Predictive Analytics:
The main techniques in healthcare predictive analytics include ML, data mining, statistical modeling, and AI.
1- Data Collection and Analysis:
Data collection is the first step in predictive analytics. In healthcare, this means gathering information from various sources such as electronic health records (EHRs), medical devices, patient surveys, and lab results.
- Analyzing hospital performance trends
- Gathering data from patient visits, medications, and procedures
- Organizing data for easier analysis
- Collecting patient health data
2- Machine Learning Algorithms:
Machine learning (ML) plays a major role in predictive analytics. It is a type of artificial intelligence that allows computers to learn from data and improve over time without being explicitly programmed.
In healthcare, ML algorithms can be trained on past patient data to predict the likelihood of certain conditions, such as heart attacks, strokes, or diabetes.
- Predicting patient health risks
- Identifying high-risk patients for early intervention
- Improving the accuracy of disease diagnosis through historical data
- Spotting trends in patient data for early intervention
Steps to Implement Predictive Analytics in Healthcare:
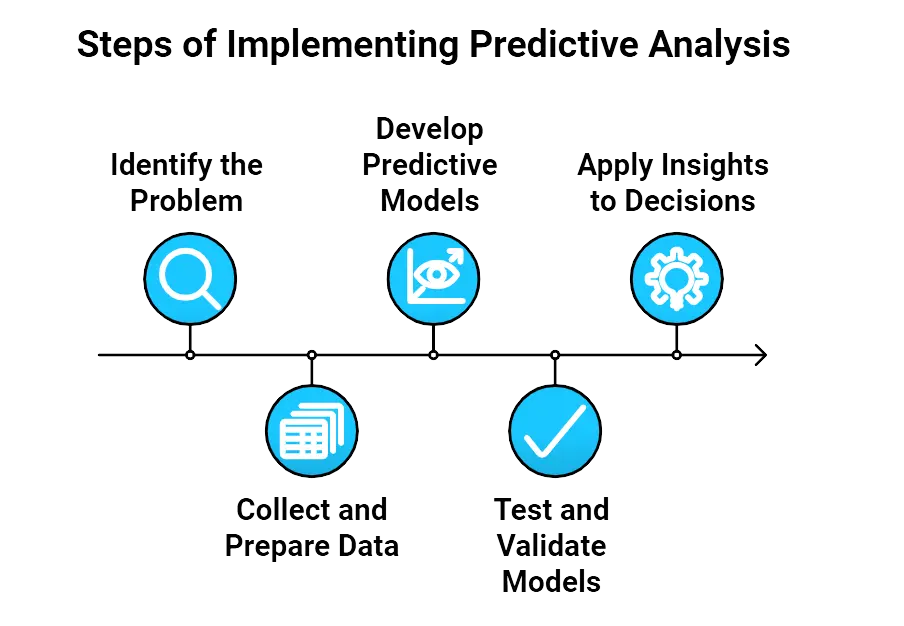
To implement predictive analytics, healthcare organizations should follow a clear set of steps.
- Define the healthcare problem to solve
- Prepare and clean the necessary data
- Build predictive models using machine learning
- Validate models to ensure predictions are accurate
- Use insights to guide clinical and operational decisions
Artificial Intelligence Predictive Analytics in Healthcare:
Artificial intelligence predictive analytics plays a key role in improving healthcare operations. AI and predictive analytics allow healthcare providers to make more accurate predictions by analyzing vast amounts of data faster and more efficiently than humans can. AI systems use algorithms to find patterns in data that might not be obvious to human analysts.
One example of AI in healthcare is the Medical AI Chatbot, which can analyze patient data and provide insights, offer medical advice, or even assist in diagnosing conditions based on patterns it identifies in patient histories.
Role of AI and Predictive Analytics:
AI and predictive analytics support by using advanced algorithms to process large datasets quickly. In healthcare, AI can analyze patient information, medical histories, and even lifestyle factors to predict future health risks.
AI predictive analytics enhances clinical decision-making by providing real-time insights and recommendations based on patient data.
- Identifying disease patterns in patient data
- Predicting the likelihood of future health issues
- Analyzing medical imaging data for early diagnosis
- Making better use of electronic health records
For instance, AI-powered tools can analyze medical imaging data to predict the development of diseases like cancer or cardiovascular conditions.
How AI and Predictive Analytics Improve Healthcare:
AI and predictive analytics are more effective in improving the accuracy of predictions. Traditional statistical methods might miss subtle patterns or correlations, but AI can handle complex data more efficiently.
- Continuous patient monitoring through AI
- Predicting outcomes based on patient data and genetics
- Reducing errors in diagnosis and treatment planning
For example, AI Medical History can analyze a patient’s entire medical history, lifestyle choices, and genetic information to make predictions about their health.
AI Predictive Analytics in Healthcare: Outcomes
AI models can be trained to predict various health outcomes, such as the likelihood of patients developing chronic conditions like diabetes, hypertension, or respiratory diseases.
AI predictive analytics in healthcare have been used to monitor patients for warning signs of respiratory failure, allowing for timely interventions.
- Forecasting heart disease risk based on patient data
- Early detection of high-risk patients
- Offering personalized preventive measures based on AI insights
- Predicting chronic diseases like diabetes
For example, AI algorithms can predict the chances of a patient developing heart disease based on factors like cholesterol levels, family history, and lifestyle habits.
AI Predictive Analytics Examples:
AI predictive analytics in healthcare is increasingly being used in various medical applications to help improve patient care, reduce costs, and make healthcare processes more efficient. AI predictive analytics tools have increased customer engagement by 40% in financial services, a trend extending to healthcare through personalized patient interactions.
One example is how AI predictive analytics can predict disease outbreaks by analyzing patterns in data from hospitals, health departments, and even social media.
Example | Description | Benefits |
---|---|---|
Predicting Disease Outbreaks | Uses data to foresee potential disease outbreaks, allowing quicker response. |
|
Predicting Patient Readmission | Helps hospitals know which patients might need readmission, improving care. |
|
Identifying High-Risk | Can identify patients who may develop serious conditions, allowing early intervention. |
|
Treatment Predictions | Predicts which treatments will work best for each patient based on condition. |
|
Predictive Analytics and Healthcare Examples:
Predictive analytics in healthcare is being used widely to help predict patient outcomes and manage resources more effectively.
Another example is in the area of chronic disease management. AI can monitor patients with diseases like asthma, diabetes, or hypertension. By analyzing their daily data, AI and predictive analytics can predict when their condition may worsen, helping healthcare providers take preventive actions before it’s too late.
- Optimizing emergency department staffing and resources.
- Predicting patient medication non-adherence.
- Assigning health risk scores for preventive care.
- Predicting mental health crises for timely interventions.
Case Studies of AI Predictive Analytics Integration in Healthcare:
AI predictive analytics have achieved up to 91.2% accuracy in predicting high-cost claimants, enabling targeted care management. These models can analyze a wide range of data, including lab results, vitals, and historical patient information.
One example is the Cleveland Clinic, which uses AI predictive analytic to predict which patients are most likely to develop heart disease. By analyzing patient data, the clinic can provide targeted treatments to those most at risk, potentially saving lives and reducing long-term healthcare costs.
Another example is the Mount Sinai Health System in New York. They use AI and predictive analytics to improve patient care by predicting which patients might need intensive care. AI systems help doctors prioritize resources, ensuring that critical patients receive the care they need without overburdening the hospital staff.
The Pros and Cons of Predictive Analytics in Healthcare:
Predictive analytics brings several benefits to healthcare. It helps doctors and nurses make better decisions by predicting health risks and suggesting the best course of action.
Pros | Cons |
---|---|
Improves diagnostic accuracy | Privacy concerns with patient data |
Helps with resource allocation | Risk of relying too much on technology |
Enhances patient monitoring | Requires large amounts of data |
Improves decision-making | Potential for model bias |
Reduces healthcare costs | High implementation and maintenance costs |
Increases efficiency in healthcare systems | Potential for errors in AI models |
Aids in personalized treatment plans | Ethical concerns about data use |
Advantages of Using Predictive Analytics in Healthcare:
For patients with chronic illnesses, predictive analytics in healthcare, along with remote patient monitoring, can track their health in real-time, allowing healthcare providers to intervene before problems get worse. By monitoring signs and symptoms from a distance, healthcare teams can spot potential issues early and take timely action.
- Early detection of diseases like cancer and diabetes.
- Improved patient outcomes with timely interventions.
- Remote monitoring for chronic illness management.
- Better hospital resource management with predictions.
Challenges of Predictive Analytics in Healthcare:
While predictive analytics offers many benefits, there are also challenges. One major issue is the accuracy of predictions. AI and predictive analytics in healthcare systems rely on large amounts of data, but if the data is incomplete or biased, predictions can be incorrect.
For example, if a system is trained on data from only one demographic group, it may not be accurate for other groups. This can lead to biased outcomes that affect patient care.
- Data accuracy concerns in predictive models.
- Bias in AI predictions if data is incomplete.
- High costs of implementing AI systems.
- Privacy and security risks with patient data.
Healthcare Organization Using AI in Data Analytics:
Healthcare organizations using AI in data analytics are increasing in number to improve patient care and optimize operations. AI helps doctors, nurses, and administrators make better decisions by analyzing large amounts of data more quickly than a human could.
Predictive analytics integrates well in hospitals and clinics to collect data from various sources such as patient records, lab results, and medical imaging.
AI predictive analytics tools process this data to uncover patterns or forecast health risks. This integration helps healthcare providers make informed decisions, improve patient outcomes, and enhance the overall efficiency of healthcare systems.
Challenges for Healthcare Organizations:
- AI systems need large amounts of accurate data to be useful
- There may be concerns about patient privacy and data security
- Integrating AI tools into existing healthcare systems can be difficult
- The cost of implementing AI in healthcare can be high
Examples of Healthcare Organizations Using AI:
Predictive analytics and healthcare are increasingly intertwined as several hospitals and clinics adopt AI to enhance healthcare services. By integrating AI into data analytics processes, hospitals can deliver improved services, minimize errors, and enhance results.
AI Predictive Analytics Applications:
- Mayo Clinic: Uses AI for analyzing medical records and improving diagnosis accuracy.
- Mount Sinai Health System: Implements AI in medical imaging to detect diseases like cancer early.
- Johns Hopkins Hospital: Uses AI to predict patient needs and monitor vital signs.
Smart Medical History in Healthcare:
Smart Medical History is an AI medical history software that collects and analyzes a patient’s medical history, lifestyle choices, and genetic data to provide real-time health insights. This tool allows healthcare providers to gather information efficiently, helping them make informed decisions about patient care.
By integrating Enterprise AI Solutions, healthcare organizations can scale this technology across multiple systems, streamlining workflows and enhancing patient care through accurate, data-driven decisions.
- Data Collection: The chatbot gathers detailed patient history, reducing the need for lengthy manual forms.
- Health Insights: AI analyzes data to predict risks and suggest personalized treatment plans.
- Improved Patient Engagement: Patients can easily provide updates on their health, improving communication with healthcare providers.
- Reduced Administrative Burden: Healthcare providers spend less time gathering and inputting patient data, freeing up resources for direct patient care.
The Future of Predictive Analytics in Healthcare:
As we look into the future, AI and predictive analytics will continue to play an important role in healthcare. With more data being collected every day, healthcare systems will increasingly rely on these tools to predict patient needs and outcomes.
Predictive analytics is changing patient care by improving historical data, patient health records, and advanced algorithms. This integration enables healthcare professionals to anticipate potential health issues before they occur.
- Predictive models will help doctors identify health risks early.
- AI and machine learning will become more common in hospitals.
- Healthcare systems will rely on predictive analytics for better decision-making.
- Health predictions will be based on both patient data and trends.
This approach will help doctors make better decisions and provide more accurate care plans, improving overall patient outcomes.
Emerging Trends in Predictive Healthcare Analytics:
New trends in predictive analytics and healthcare, as well as the integration of AI and predictive analytics, are reshaping the approach to patient care. One significant trend is the adoption of wearable devices that collect real-time health data, such as heart rate, sleep patterns, and physical activity.
- Wearable devices are providing real-time health data to doctors.
- AI can predict health risks through electronic health records.
- Predictive models will become more accurate with better data.
- The use of patient data will improve care and reduce hospital visits.
Another trend is the growing use of EHR systems combined with predictive analytics. By analyzing vast amounts of patient data, AI tools can identify patterns and predict health risks more accurately.
Ethical Considerations in Healthcare Predictive Analytics:
As healthcare adopts predictive analytics, there are ethical concerns to consider. In the realm of predictive analytics and healthcare, one major issue is data security and privacy. With sensitive health information being collected and analyzed, it’s crucial to ensure that patients’ details are protected.
Another concern is fairness. Predictive models may unintentionally reflect biases based on factors like age, gender, or race. If these biases are not addressed, certain groups may receive less accurate predictions.
- Protecting patient data is essential to ethical predictive analytics.
- Bias in predictive models can lead to unfair healthcare outcomes.
- Regulations are needed to ensure data is used correctly and securely.
- Healthcare systems must address ethical challenges to ensure fairness.
Addressing Bias in Predictive Models:
One of the most pressing ethical challenges in predictive analytics in heathcare is the issue of bias. Predictive models often use historical data to make predictions, and if that data contains biases—such as underrepresentation of certain groups. This can result in certain patients being overlooked or misdiagnosed.
To address this, healthcare organizations need to make sure that the data used in these models is diverse and representative of the entire population.
- Ensuring diverse data sets is key to eliminating bias.
- Regular reviews of predictive models can help spot bias.
- Ethical oversight is needed to improve the fairness of AI tools.
Subscribe to our Newsletter!
Subscribe our Newsletter to stay updated
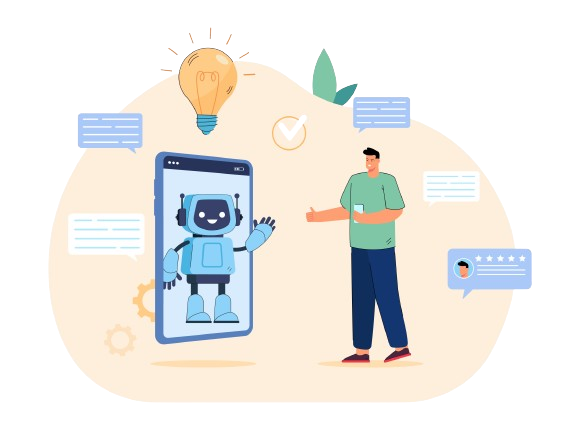
Conclusion:
The impact of predictive analytics on healthcare is significant and will continue to grow in the coming years.
- Predictive analytics improves care and reduces healthcare costs.
- AI and predictive analytic tools help doctors catch health issues before they worsen.
- Healthcare systems will become more effective with data-driven decisions.
- Predictive analytics will play a central role in the future of healthcare.
Predictive analytics in healthcare also holds the promise of reducing inefficiencies and improving the overall healthcare experience for both patients and providers. By using data to guide decision-making, healthcare systems can be more efficient and effective.
Read More Blogs
See what’s trending in the medical world with our blogs.